What is AI?
There are different ways one can view AI. Here are a few views I resonate with. These differing perceptions are somewhat orthogonal.
- It is a field of study and research on how to make machines more intelligent.
- It is a branch of computer science.
- It is a set of technologies and processes to mimic human level intelligence in machines
In short, AI is an umbrella term used to cover different approaches to get machines to learn, reason and provide intelligence as close to human intelligence as possible.
To avoid ambiguity, let us talk about AI technology and AI applications. AI Technology consists of a set of methods to derive intelligence from different types of data (text, numbers, video, audio, images and signals of various kinds).
Applied AI is the application of AI technologies to make applications, and devices smarter. For example using audio signals to recognize speech and convert it to text is an application of AI that most of us can relate to. Identifying objects in an image, recognizing digits, detecting anomalies, making predictions are some of the more popular applications of AI.
AI technologies have been evolving over a period of time. In the early stages (late 50s and 60s) AI was all about domain knowledge and rule based inferences. As the limitations of these approaches unraveled, we slowly started moving towards statistical methods driven by data. Machine learning a sub-field of artificial intelligence is currently based on two major approaches - statistical machine learning and deep learning.
Statistical machine learning provides different techniques, algorithms and models for processing data and deriving insights. Deep learning is currently based on artificial neural networks. A wide variety of neural networks have evolved to solve different problems. Artificial neural networks get their name because they are made up of artificial neurons which model a part of the behavior of the neurons in human brains.
AI is an emerging field of study and research. So we need to temper our expectations of what it can do. There are some narrow areas where AI has advanced swiftly and effectively. These include playing games that humans were good at (Chess, Go and others), recognizing speech, translating languages, predicting customer behavior, detecting fraud and others. Before AI techniques arrived at the scene, attempts were being made to use rule based systems to perform some of these tasks.
AI is getting good at solving a new class of problems that humans can solve, but finds difficult to automate. For example, we can recognize handwritten digits or recognize objects in images. But we're not able to instruct a machine on how to do it. Here is where Machine Learning helps. You give these AI systems a large amount of sample data labelled with the objects contained in them. AI applications find patterns in data and find correlations between inputs and outputs and create models from the training set. With several iterations, we improve these models and deploy them as solutions.
Science is very data driven. Many branches of Science gather large amounts of empirical data and draw inferences from them. New developments in sensors make data gathering easier but the resulting information is increasing in volume, and velocity. Machine learning techniques are increasingly becoming useful tools for handling more data without explicit programming.
"Today’s AI commonly works by starting from massive data sets, from which it figures out its own strategies to solve a problem or make a prediction—rather than rely on humans explicitly programming it how to reach a conclusion. The results are an array of innovative applications.
AI technology is increasingly used to open up new horizons for scientists and researchers. At the University of Chicago, researchers are using it for everything from scanning the skies for supernovae to finding new drugs from millions of potential combinations and developing a deeper understanding of the complex phenomena underlying the Earth’s climate."[1]
Big Data was one of the primary drivers for the adoption of ML techniques. The term Big Data is a bit misleading. Big data is volume, velocity (the speed with which data arrives) and variety (the types of data). For example in the early stages of automation we had large amounts of numerical data. Now we have data in other forms like spreadsheets, text, audio, images, video and various forms of sensor data. Along with the growth of big data, we are seeing growth in various techniques for handling data which includes distributed computing techniques, reduction techniques and analysis techniques driven by Machine Learning approaches and algorithms.
AI is finding use in many cross-functional areas for business as well as specific uses in various industries. Shivon Zillis did a great job of creating a market map of the industry. [2]
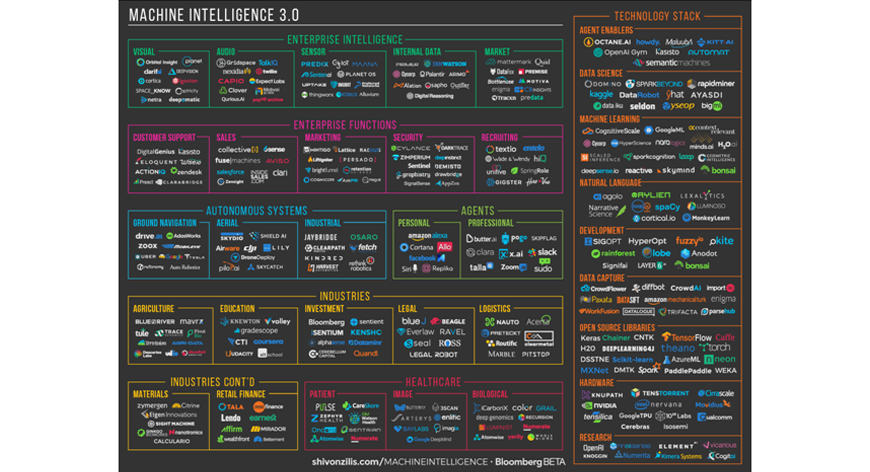
There are several functional AI services used across industries. These include:
1. Prediction Engines
2. Classification Systems
3. Clustering techniques
4. Anomaly Detection systems
5. Recommendation systems
Wikipedia AI page shows a growing list of industries and applications.[3]
AI is an emerging technology space with infinite possibilities. But it is not without problems. So tread carefully when you try to adopt it for your business. Here are a few things to pay attention to.
- Good AI skills are scarce. Some of the best resources are hired by the top software companies like Amazon, Google, Facebook, Microsoft, Twitter and others. They do research, build models with large amounts of data and derive many benefits from building their own applications. They do provide services for others to use through the cloud.
- Many wonderful stories you hear about AI are in the research stage. Check adoption rates and successful projects before you make a commitment to using these techniques.
- Right now AI is a black box. There are movements like Explainable AI to fix the mystery behind how it works. They are in a nascent stage.
- Ethical use of AI is a big concern. The ethics of proper use of AI by governments and intelligence agencies is a hot topic of discussion.
- People are afraid that AI may replace people. I think it is an overreaction at this point in time. While AI may reduce the need for certain tasks in a job, complete elimination of jobs will take a while.
AI is currently very narrow in scope. It has a name ANI - artificial narrow intelligence. The AI that can beat a gamer in Go is not suitable (yet) to perform fraud detection. ANI applications are still silos. There are a few signs of Transfer Learning (ability to take models from one domain and reuse them in other domains) but it is not widely available yet.
We need to start worrying when we get to AGI - artificial general intelligence. Estimates vary about our ability to produce AGI. Some think it may be as early as five years and others feel that it will over 50 years. The concept of AGI itself range from reusable intelligence models to human level AI.
I would like to conclude by pointing out that like every other technology that came before it, AI has many exciting possibilities. These will not only stretch our imagination but also provide a better understanding of how humans learn and think.
Reference(s)
- https://news.uchicago.edu/story/how-ai-could-change-science
- Shivon Zilis - Machine Intelligence http://bit.ly/1O7z7lx
- Applications of artificial intelligence
About the Author
Dorai Thodla helps students and startups build product skills. He co-founded 4 software companies - two in India and two in the USA. His companies build Information Assistants, a collection of tools for gathering and analyzing information. He conducts seminars and workshops on AI and its impact on the future of work.